Introduction
The purpose of using deconvolution is to convert the measured pressure data response from a variable rate test into a response that the reservoir would exhibit if the wells were produced at a constant rate for the entire production history. This paper illustrates deconvolution abilities in analyzing real well-test data in one of Iran's southwest oil fields. Deconvolution is an interpretation method that can extract the measured response in the form of a constant drawdown rate with a duration equal to the total test duration that has emerged with the development of robust pressure/rate deconvolution algorithms (von Schroeter et al. 2001, 2002, 2004; Levitan 2005; Levitan et al.2006, 2012; Ilk et al. 2005, 2006, 2007). Deconvolution is a data processing and is independent of the reservoir type. It works as long as the system is linear or close to linear. For gas reservoirs, we have to use single-phase pseudo pressure or 2-phase pseudo pressure below the dew point pressure.
The deconvolution algorithm considered in this paper is based on the Total Least Square method first described by von Schroeter et al. (2001). This algorithm with some variations is used in most commercial well-test analysis software.
The paper is organized as follows: step-by-step progress of deconvolution algorithms is presented. In the second part, we compare the performance of deconvolution and conventional methods by considering a real well-test model. The last part is dedicated to the discussions and main conclusions.
Summary
Deconvolution is a mathematical tool to extract the unit rate function from the pressure data in multi-rate tests. This Unit rate function is the reservoir type curve; it facilitates the identification of the reservoir model. The foundation of deconvolution in the well-test analysis is Duhamel’s principle which is an extension of the superposition principle. The pressure rate deconvolution goal is to estimate the constant rate of reservoir response using the observed measurements of pressure drop and flow rate.
In a linear system, the pressure drop at the well bore during a variable rate test is given by the convolution integral. Mathematically convolution integral is a special case of the first-kind Volterra equation that is ill-posed and is wildly known for solving time-dependent boundary value problems.
P (t) = Pi - ∫(𝜏)𝑔(𝑡−𝜏) 𝑑𝜏𝑡0 (1)
Here, q (t) is the well rate, P (t) is the well bottom hole pressure, Pi is the initial reservoir pressure and g denotes the reservoir response which is the ordinary time derivative of the rate-normalized wellbore pressure drop:
tg (t) = 𝑑 Δ𝑝𝑢(𝑡)𝑑 𝐿𝑛𝑡 (2)
The response of any dynamic model to external changes is called the reservoir response. The impulse response is the output of the time domain systems that received the signal as input.
Van Everdingen & Hurst (1949) introduced Duhamel’s principle into the well-test analysis. They used Laplace transformations to linearize the diffusivity equation with simplifying assumptions such as aquifer homogeneity and elementary reservoir-aquifer geometries. The linearity of the diffusivity equation makes it possible to use Duhamel’s principle.
Eq. (1) is valid for the condition that the wellbore storage coefficient and skin are constant during the entire well test period. Some degree of inconsistency exists dealing with real test data. Levitan (2005) solved this problem by applying the current deconvolution algorithm based on Eq. (1) not only to all the pressure data at once but also to pressure data from individual flow periods. The nonlinear behavior of gas and oil-saturated reservoirs makes erroneous results in the application of deconvolution. Levitan and Wilson (2012) suggested an approach for upgrading deconvolution for use in nonlinear mode. At high pressures (above 3000 psi), gas property changes very little and the behavior is linear. When the reservoir pressure declines significantly below 3000 psi, they used pseudo pressure and material balance pseudo time for the pressure and rate data. By using the pseudo pressure transform, the linearized deconvolution formulation becomes as follows:
m (t) = mi - ∫𝑞(𝜏)𝑑 𝑚𝑢(𝑡−𝜏)𝑑 𝑡𝑡0 dτ (3)
Here, q(t) is the well rate, m(t) is the bottom hole pseudo pressure, and mi is the initial reservoir pseudo pressure. Pseudo-time transformation is used to linearize the gas flow problem in addition to pseudo-pressure transform.
ta = Cti μgi ∫𝑑𝜏𝐶𝑡(𝑝)μ𝑔(𝑝)𝑡𝑡𝑖 (4)
Error minimization
Analytical expression based on a simple Gaussian model is used for the error measurements in both pressure and rate data. Regularization based on a measure of the total curvature of its graph is also used to maintain a degree of smoothness in the solution. Total Least Square formulation is applied for computing the errors in the rate and pressure data (von Schroeter 2002, 2004; Gloub and van Loan 1996; Björck 1996). So, the deconvolution problem is formulated as an unconstrained nonlinear minimization which the objective function defined as (von Schroeter 2002, 2004):
E = ǁp0 Ym – p – C (z)yǁ22 + 𝜗ǁy - qǁ22 + 𝜆ǁDz - Kǁ22 (5)
The first term is pressure match (ɛ) and the second one is the rate match (𝛿). A good deconvolution is obtained by the smallest value of ɛ and 𝛿. Ym is a vector of size m that each component equal to 1 and 𝜗= 𝑁𝑚 ǁ𝛥𝑝ǁ22ǁ𝑞ǁ22 and 𝜆 = ǁ𝛥𝑝ǁ22𝑚 are the rate match and regularization parameter respectively. Where Δp is the vector of pressure drop data, q is the vector of measured rate data, m is the number of pressure data points and N is the number of flow periods.
Deconvolution implementation
In this section, we illustrate the main advantage of deconvolution, the large radius of investigation, by use of some real data analysis from one of Iran's southwest oil fields which allows for detecting boundaries and connectives that could not be seen in conventional analysis.
Field data
In this study, the pressure data was taken by drill stem test for an exploration well in one of Iran's southwest oil fields. Figure 1 and Figure 2 present the pressure response of the reservoir that is obtained from conventional and deconvolution methods. One main advantage of deconvolution is developing additional information from pressure transient behavior by increasing the radius of investigation, which allows seeing reservoir features that is not possible by using individual flow periods analysis.
Well Test A:
This drill stem test (DST) was conducted in a vertical well. The initial pressure obtained from DST is 9177 psi and the reservoir is infinite acting. In general, the duration of this test is 126.4 hours and includes three build-up periods. As shown in Figure 1, the buildup test with 33.4 hours
duration has been selected for conventional analysis.
Figure 2 shows a comparison between conventional and deconvolution results. The pressure derivatives for the first, second, and third build-up periods show some shift that could be caused by some error in flow rate measurements. The diagnostic derivative plot of the second set of PBU pressure data (as the conventional method), in contrast with the deconvolution method, cannot show late time flow regime. The yellow curve is deconvolved and the green one (main build-up period) is the conventional result. The deconvolution response clearly shows all flow regimes correctly. Figure 2 shows that the deconvolution algorithm completely and accurately recovers the response function.
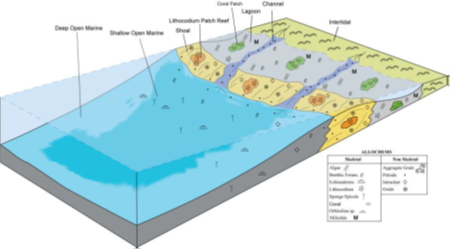
Figure 2. Comparison of Deconvolution and Conventional Derivative Analyzed Model
For verification of the deconvolution result, there are some methods: 1) the generation of pressure history same as actual pressure history. 2) the ability to match a deconvolved with conventional derivatives. Therefore, important parameters resulting from the two methods are almost the same. Just radius of the investigation for the deconvolution method equals to total test duration and for this test radius of investigation by the deconvolution method is 1446.2 (ft) and by the conventional method is 892.3 (ft). Permeability for deconvolution and conventional analysis is 19 and 17.5 (md). Skin value for deconvolution and conventional analysis is 5.7 and 5.42.
Conclusions
We have discussed the advantages of the deconvolution method in transforming multi-rate data into constant-rate draw-down data. This transformation combines all the multi-rate recorded and effectively increases the radius of investigation of recorded data. Large radius of investigation allows for better analyze sectional zones and boundaries which conventional methods cannot observe and analyze. The practical advantage of the deconvolution approach is shown via three comparative well-test studies with both the convolution and deconvolution methods. It is therefore beneficial to use all the flow periods recorded during well-test operations and convert them to equivalent constant-rate data via the deconvolution method. This method increases the radius of investigation of recorded data and better captures the geological events and boundaries that otherwise we would never observe by conventional pressure derivative diagnostic method.
Acknowledgments
The author would like to thank the Managing Director, Management Committee, and Subsurface Department of Phase 11 for their support and permission to publish this paper.
References
- Gringarten AC. Practical Use of Well-Test Deconvolution. 2010. SPE: Society of Petroleum Engineers.
- Ilk D, Valko PP, Blasingame TA. A Deconvolution Method Based on Cumulative Production for Continuously Measured Flowrate and Pressure Data. 2005. SPE: Society of Petroleum Engineers.
- Levitan MM, Crawford GE, Hardwick A. Practical Considerations for Pressure-Rate Deconvolution of Well Test Data. 2006. SPE: Society of Petroleum Engineers.
- Levitan MM, Wilson MR. Deconvolution of Pressure and Rate Data from Gas Reservoirs with Significant Pressure Depletion. 2005. SPE: Society of Petroleum Engineers.
- Levitan MM. Practical Application of Pressure-Rate Deconvolution to Analysis of Real Well Tests. 2012. SPE: Society of Petroleum Engineers.
- Onur M, Cinar M, Ilk D, Valko PP, Blasingame TA, Hegeman PS. An Investigation of Recent Deconvolution Methods for Well-Test Data Analysis. 2007. SPE: Society of Petroleum Engineers.
- von Schroeter T, Hollaender F, Gringarten AC. Analysis of Well Test Data From Permanent Downhole Gauges by Deconvolution. 2002. SPE: Society of Petroleum Engineers.
- von Schroeter T, Hollaender F, Gringarten AC. Deconvolution of Well Test Data as a Nonlinear Total Least Squares Problem. 2001. SPE: Society of Petroleum Engineers.
- von Schroeter T, Hollaender F, Gringarten AC. Deconvolution of Well-Test Data as a Nonlinear Total Least-Square Problem. 2004. SPE: Society of Petroleum Engineers.